For the first time, NOAA is launching a national rip current forecast model, aimed at saving lives of beach-goers around the country. This new model can predict the hourly probability of rip currents along U.S. beaches up to six days out.
Extensive collaborations apply machine learning algorithms to identify protected species from images and acoustic recordings. NOAA Fisheries.
In an ocean world where things like climate change and overfishing have the ability to drastically alter the functionality of entire ecosystems, having a stethoscope to detect signs of major issues could really come in handy to marine resource managers. National Marine Sanctuaries.
NIDIS (National Integrated Drought Information System) uses machine learning to develop a model to recreate the US Drought Monitor using input drought indicator products. This a collaboration with NASA's Earth Sciences Division at Goddard Space Flight Center, who is conducting the research using NOAA funds from NIDIS. Lead, Molly Woloszyn, NIDIS.
A successful NOAA study applies deep machine learning to analyze a large passive acoustic dataset and identify highly variable humpback whale sounds across broad spatial and temporal scales. Lead: Carrie Wall Bell, NCEI/CIRES.
NOAA teams are training AI tools to distinguish a seal from a rock and a whale’s whistle from a dredging machine’s squeak as they seek to understand the marine mammals’ behavior and help them survive amid melting ice and increasing human activity. Lead: Erin Moreland, NMFS.
A new machine-learning tool developed by NOAA NCEI and affiliates in Boulder, Colorado, is giving space weather forecasters an advantage. Lead: Dan Seaton, NCEI/CIRES.
NOAA affiliate scientists used AI to reduce by 93 percent the time they spend on analyzing the sounds of endangered beluga whales for identification. Lead: Manuel Castellote, NMFS.
NCAI collaborative challenge projects

NCAI & Water Column Sonar
Goal: Develop an AI-ready Water Column Sonar Data Lake to "find little fish in big data". Status: (a) Zarr encoders in development; (b) exploring NCCF requirements and Infrastructure as Code specifications.
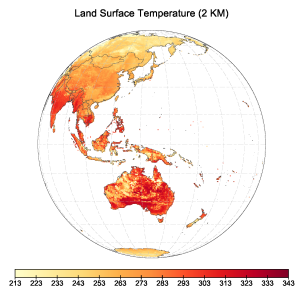
Land Surface Temperature
Goal: Explore the use of AI on Himawari-8 and GOES-13 imager data to develop improved Land Surface Temperature (LST) estimates. Status: Developing simulated training data for upcoming Neural Network model.

Magnetic Modeling (Dst)
Goal: Developing a real-time space weather Disturbance Storm Index (Dst) for enhanced magnetic navigation. Status: X-prize completed; exploring R2O paths and developing a peer-reviewed publication.